2023 is the year of AI, but how do AI and Large Language Models work is the question being asked. Generative AI has become one of the hottest topics in tech, potentially impacting almost every industry in some way, shape or form. This evolution is driven by statistics that show that within the next two years, as much as 10% of all data produced globally will be done so by AI.
This offers organisations the potential to analyse themselves, their market and their customer base in greater detail, and potentially achieve a significant cost benefit, especially if they adopt LLMs (Large Language Models) sooner rather than later.
Following the Google I/O conference, in which the tech company revealed big plans for its AI, in this blog, Matt Penton, Head of Data and Analytics at Appsbroker, offers his insight into why we should all be cautiously optimistic about the Future of AI.
What is a LLM and how do they work?
A Large Language Model (LLM) is trained on huge amounts of data, typically text-based data, structured or unstructured, that can utilise pre-trained models. LLMs have existed for many years, most commonly used in call centres for call agents and inference pattern matching. However, the sophistication of these large language models has come on leaps and bounds in more recent times, including high-profile open-source technologies like Alexa or Google Assist.
Traditionally when you build up a LLM you would train it with your data, so it can recognise your text, then learn and adapt, also known as self-recognition. Alternatively, you could use a feedback model. These work well out of the box and can generate text based on what it has learned across the internet. Generally, these have a very strong understanding of certain things without ever having to explain them.
Can AI be used to increase productivity?
Whilst generative AI may be used to reduce call centre headcount, it’s not marketed as such. A common positive example of integrating programs such as ChatGPT and Bard into businesses is the salon model, where an AI can answer calls and talk to customers automatically, so the hairdressers can focus their efforts on cutting hair. They’ve even put humorisms (umms, ahhhs and breaths) in the AI’s speech to enable more human-like responses. Google Assistant ran a similar test, where a Generative AI rang a salon to book an appointment. See the results:
Are LLMs reliable?
When asking how AI and Large Language Models work, a lot of the questions often challenge the reliability of these models. In that sense, the answer comes down to trust, and the application of a good amount of caution. For example, AI models have only a limited ability to track intent track. So, if you change the subject halfway through a conversation with an AI, it finds it difficult to understand and re-contextualise in the same way a human could.
Generative AI can be used to cut down on the more mundane day-to-day tasks, freeing up as much as 30% of a person’s week to leave time for more important things. From planning meetings to writing presentations, it’s likely to change the working day for most people.
What is the difference between Bard and ChatGPT?
Bard and ChatGPT operate largely on the same principles of using identical algorithms to be able to work off pre-trained massive large language models. The difference lies in the data sources that have been used to train the models which each require feedback loops – the more data they get back, the better the model becomes. And, as Bard is newer than ChatGPT, the two models offer a different type of experience.
However, one of the main benefits of Bard is that it has access to Google search data, of which there is lots. Recent records show over 99,000 Google searches are conducted every second which adds up to over 8 billion searches per day – the equivalent of more than one search for every person on Earth. That is quite the training model when you think about it.
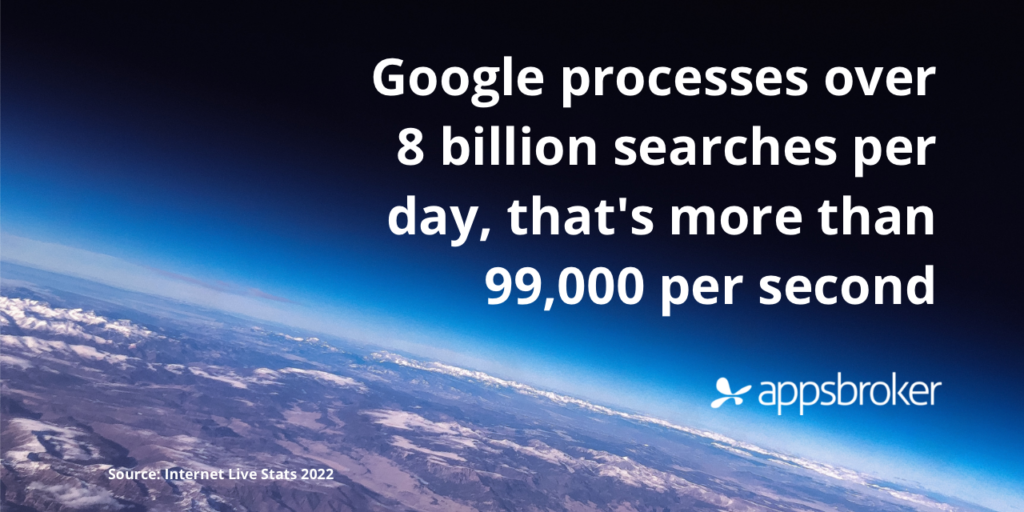
This is another great example. Bard feeds off the internet in real-time, giving users more representative and “live” results based on a dataset that is constantly being updated. ChatGPT works from existing data, of which there is a phenomenal amount, but this means users have to subsequently connect to the internet to get a more reflective response.
How can businesses use AI as a project tool?
A proven way to to find out how AI and Large Language Models work is to look at ways businesses currently factor AI into their ops through project management. Basically, wherever there is a lot of text, we can use solutions from Google and other third-party providers to provide a narrative on areas like performance, or a better understanding of a user’s requirements, which ultimately improves efficiency. And, as a priority, companies should be embedding it into customers with the correct safeguards around synthesized text and images which, if utilised correctly, could be extremely effective.
For example, the pharmaceutical industry is able to generate drug designs and optimise drug testing. In the case of covid vaccines, they can take an existing SARS vaccine and understand it to produce a drug design that will adapt as the virus evolves. That could cut research times and improve the quality of, if not save lives, worldwide.
One of the biggest enablers for data and analytics teams is being able to generate more data, which LLMs are very good at, by allowing us to synthesize events inside that data.
What caveats should people consider before using AI?
A side effect of how chatbots learn is what is known as an AI hallucination. Believe it or not, AI has been known to start making it up and then double down when you tell it it’s wrong, essentially gaslighting users.
For example, a particular journalist once asked an AI for tips for travelling in Japan with his daughter, who has Down’s Syndrome. The AI came back with some generic advice for travelling with a disabled child, heavy on tips referring to wheelchair accessibility, which, of course, is highly presumptuous. Here, the AI didn’t understand the context of Down’s Syndrome. The AI also warned that Britons needed to apply for a visa to travel to Japan, which is technically not true. The AI, when asked, also gave a generic response on eating out anywhere with children, and concluded with the advice, ‘Be sure to tip your server’. As the Japanese will remind you, tipping is not customary in their country, and is more often than not seen as extremely offensive.
The others are scaling and cost. Much like BigQuery, each time you search for results, you are spending money. We are already seeing this with online AI generators that provide a certain amount of free credits to be spent on generating content. Each search requires access to information stored on a server, which in turn uses energy and other associated costs. So it’s important to set a budget and define the most relevant searches to help control spending.
And finally, explainability. Take the finance industry as an example. If a customer is refused credit by a lender, they’re able to challenge the decision and ask why. But with an AI model, you may not get an answer because you’re not privy to the algorithms. Put simply, you can’t see how a decision was reached and may be churning out inaccurate results. This scenario is what we would call a Black Box Model and wouldn’t be an appropriate use of AI and ML. And now we see why responsible safeguarding measures need to be put in place both commercially and socially. Just because an AI model stated findings as fact, doesn’t automatically mean it’s correct.
Are there any cases where AI can be a force for good?
Ultimately, when we ask how AI and Large Language Models work, we look at what purpose it truly serves. And, like every new piece of technology, it’s important to think about the impact and align them with commercial or social responsibilities. And in that way, we’re able to recognise where AI is most likely to help, and in turn, we can train AI models to get better at responding.
At the moment we’re trialling Bard, which isn’t technically a LLM, as a use case for analysing the transcripts of phone calls in a regulated industry to watch for insider dealing, anti-corruption or, bribery. It can consume thousands of pages at lightning speed, and provide a summary of the call that flags the most commonly referenced areas. In the same way, this model could be used to help improve sales performance by offering highly-relevant products or services in real-time, as well as analysing language that a customer responds to positively and negatively.
Overall, AI does (and will continue to) make tasks quicker and easier. In situations where there might be too much information for a human to evaluate in the heat of the moment, AI can help spot patterns in large data sets like transcripts or even voice recognition. Again, with clearly-defined use cases with guardrails around them for security, privacy and data protection reasons.